Article
Why is data anonymization so important?
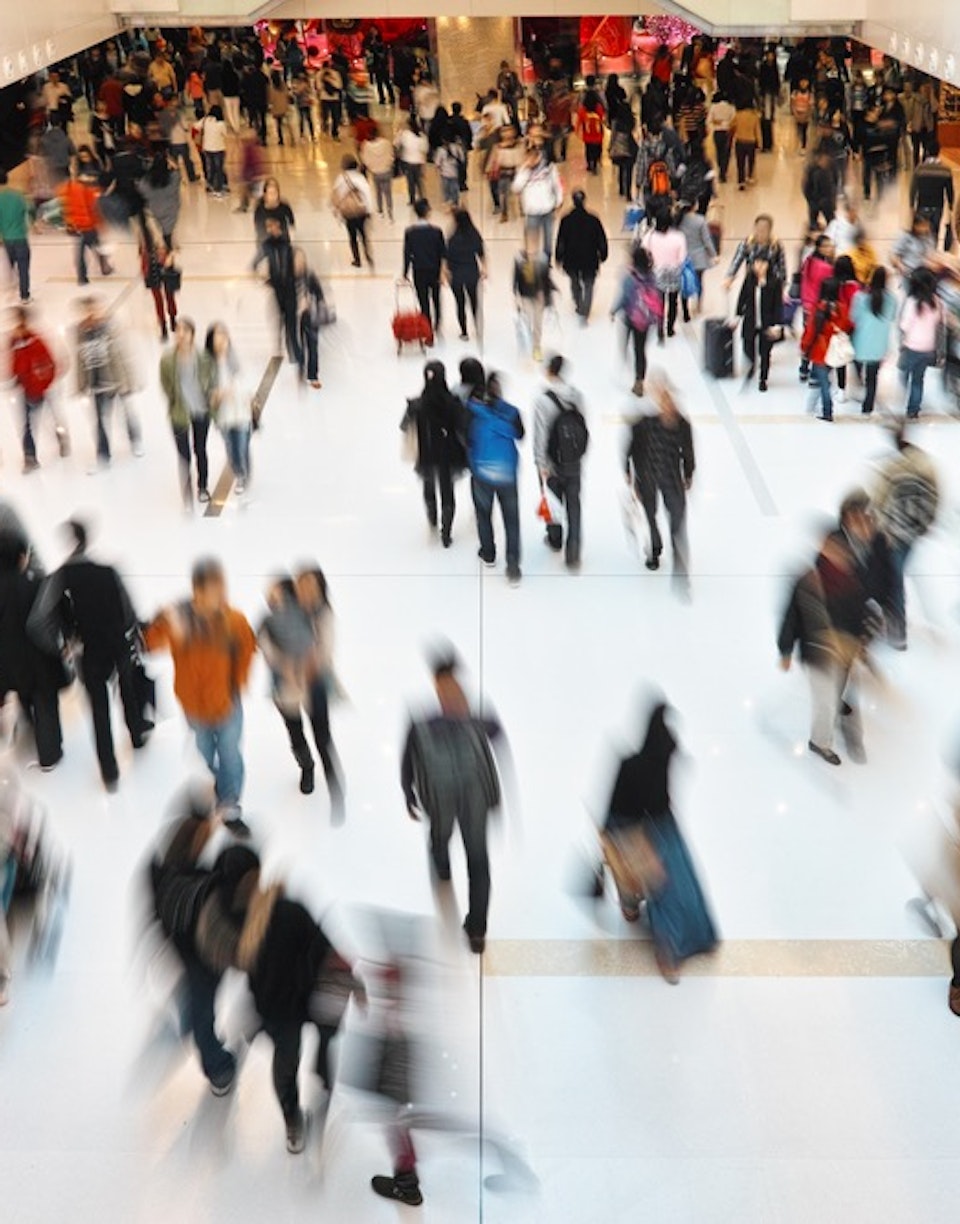
Guaranteed privacy requires data anonymization and eliminating the possibility of reverse engineering to recover data
The other day, when discussing innovation in the health sector with a client, they mentioned that they have access to a powerful database containing a vast amount of patient information. This access is obviously restricted to certain companies in order to carry out specific R&D projects for public purposes. These projects leave behind a significant trail in order to ensure data confidentiality.
The first question that came to mind was: “What steps do you take to anonymize this data and respect patient privacy?” I was told that they remove patient names and certain private information, such as phone numbers and addresses.
This is a step, without a doubt, but my subconscious quickly pictured my eight-year-old son doing reverse engineering tasks, as if undertaking a puzzle. I imagined him hard at work as he identified relationships between heart rates, ages and electrocardiogram patterns while de-anonymizing every individual behind each case history. For a child, it is a matter of time and patience, but for a machine in this day and age, only a few electrons are required.
One of the best ways to anonymize data is to classify it according to the following categories:
Personally identifiable information: Directly identifies a specific person (name, ID card number).
Quasi-identifiable values: Information that identifies a person but is also useful for our own purposes (age, weight, height, etc.).
Confidential information: Extremely useful and valuable for our own purposes (beats per minute, electrocardiogram pattern, blood pressure, etc.).
Guaranteed privacy requires data anonymization and completely eliminating the possibility of reverse engineering to recover data. This involves performing the following tasks on all of the types of data listed above:
Personally identifiable information: It is deleted. The information is completely eliminated.
Quasi-identifiable values: The data is microperturbed and microaggregated in such a way that it is grouped into a limited number of sets and ranges. Although some of the information is lost, it is an insignificant amount for the intended purposes.
Confidential information: It is respected so the information is not lost, which is a key aspect.
And how is all of this done? With machine learning algorithms that, through existing knowledge, are able to identify the type of data and apply the necessary degree of perturbation and aggregation to ensure irreversible anonymization. These are advanced SDC (statistical disclosure) algorithms with microaggregation and microperturbation strategies.
These algorithms also have a cost. The reality is that increased anonymization makes data less useful because it becomes more aggregated and perturbed.
And if you are wondering about my client, all I can say is that data privacy is now guaranteed.